Teaching EFL/ESL Learners to Describe Data: Combining Math and English
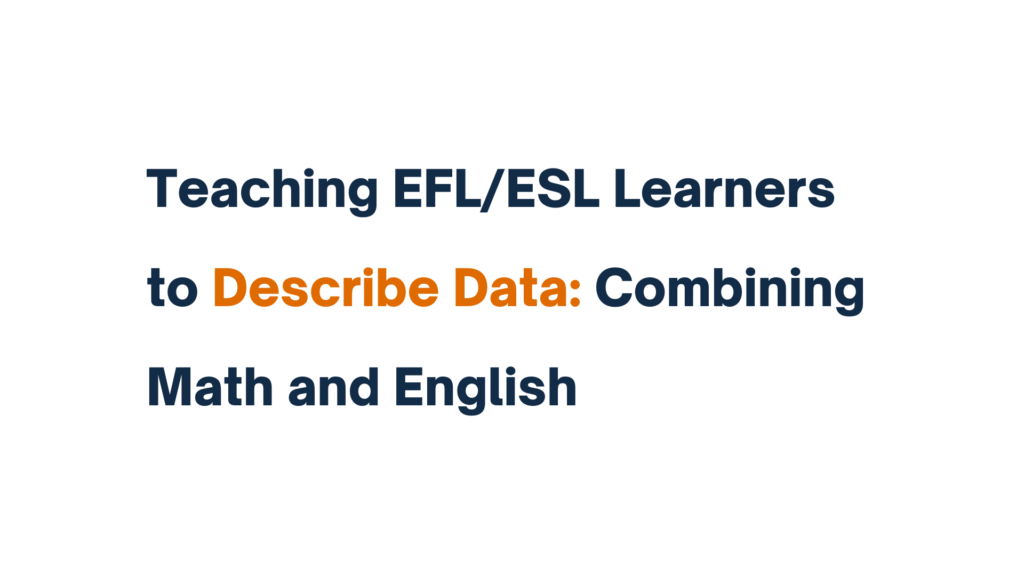
Introduction
In an increasingly data-driven world, the ability to interpret, analyze, and communicate data has become an essential skill. For EFL (English as a Foreign Language) and ESL (English as a Second Language) learners, developing these competencies is particularly challenging due to the dual requirement of mastering linguistic skills alongside basic data literacy. Teachers who focus on both linguistic and mathematical aspects can provide a holistic learning experience that prepares students for real-world contexts where data analysis and interpretation are crucial. This article explores how combining math and English language teaching can help students become proficient in describing data, a valuable skill in both academic and professional environments.
Overview of the Topic
Data literacy is the ability to understand and interpret data, a competency that has become increasingly important in today’s globalized and digitalized society. The skills involved in data literacy extend beyond merely reading numbers; they require the ability to describe and communicate insights effectively. In EFL/ESL settings, where learners often face language barriers, mastering the language functions associated with data interpretation presents a unique challenge. Many traditional language courses focus on grammar, vocabulary, and speaking skills but often overlook numeracy skills, which are vital for full participation in the modern workforce and academia.
The integration of math and English in language teaching is a pedagogical approach that aims to address this gap. By teaching students how to describe data in English, educators can help learners bridge the gap between understanding numbers and communicating insights effectively. This dual focus enhances the learner’s cognitive skills, allowing them to make connections between linguistic expression and numerical information. As EFL/ESL students increasingly encounter data in their studies, work, and everyday life, equipping them with the language needed to interpret and describe data becomes a key objective for language educators.
The Importance of Combining Math and English in Language Teaching
Integrating math into English language teaching offers several benefits, including improved critical thinking, enhanced comprehension, and better preparedness for academic and professional tasks. In traditional language classes, the emphasis is often on reading, writing, speaking, and listening. However, this approach can neglect the practical application of language skills, particularly when dealing with numerical data or quantitative information. In contrast, combining math and English in the curriculum provides a more realistic and comprehensive approach, aligning language skills with real-world needs.
Teaching data description in English involves equipping learners with the specific vocabulary and language structures needed to interpret and discuss numerical information. This includes language functions such as describing trends, comparing figures, and making generalizations. By integrating these skills into language instruction, teachers can help students gain confidence in handling data-related tasks. For instance, a student who learns how to describe a bar chart in English is better prepared to present research findings, interpret survey results, or contribute meaningfully in a business meeting. This ability to communicate data effectively is a highly valued skill across various fields, from science and technology to business and social sciences.
Moreover, the approach of combining math and English can promote deeper learning and understanding. When students are tasked with analyzing data and then using English to explain their findings, they engage in higher-order thinking. This process not only strengthens their numeracy skills but also enhances their language proficiency, as they must use precise vocabulary and grammatical structures to convey their ideas clearly. This dual development can lead to improved academic performance and greater confidence in both language and numeracy.
The Growing Need for Data Literacy in EFL/ESL Contexts
Data literacy has become a fundamental component of education worldwide, driven by the increasing availability of data in all aspects of life. In academic settings, students are often required to interpret and analyze data as part of their coursework, especially in fields such as business, economics, social sciences, and health sciences. This expectation extends to EFL/ESL learners, who must be able to handle data in English, which is the predominant language of international business and research. Without the skills to describe data accurately, EFL/ESL learners may find themselves at a disadvantage when competing for opportunities in academia and the job market.
For example, when EFL/ESL students study in English-speaking countries or enroll in international programs, they frequently encounter assignments that require data analysis and interpretation. Whether it involves summarizing survey results in a sociology class or interpreting financial data in a business course, students must be able to understand and communicate quantitative information effectively. In the professional world, this need becomes even more pronounced. Employers value candidates who can interpret data and present it clearly, using language that is precise and accessible. Thus, data literacy is not only an academic requirement but also a key employability skill.
However, teaching data literacy in EFL/ESL contexts presents unique challenges. Many learners struggle with the mathematical concepts underlying data analysis, especially if they have had limited exposure to numeracy skills in their previous education. Additionally, the specialized vocabulary and language structures needed to describe data are often unfamiliar to students, making it difficult for them to articulate their insights. Educators must therefore adopt innovative teaching strategies that integrate math and language instruction to help students overcome these barriers.
Purpose and Scope of the Article
The purpose of this article is to provide EFL/ESL educators with practical strategies and insights on how to teach learners to describe data effectively in English. The focus is on integrating math and English language skills to develop students’ abilities in data interpretation and communication. The article will cover essential aspects of teaching data literacy in language classrooms, including the role of data literacy, effective teaching strategies, key vocabulary and language functions, practical classroom activities, and methods for assessing learners’ progress.
By the end of this article, teachers will have a clear understanding of how to approach the topic of data description with their students, offering a balanced mix of theoretical background and actionable teaching techniques. Whether working with beginners or advanced learners, educators will find useful ideas and resources to help their students master the language needed to describe data accurately and confidently.
In summary, the integration of math and English in language teaching is a powerful approach that equips EFL/ESL learners with the necessary skills to interpret and describe data in real-life contexts. This article aims to serve as a comprehensive guide for teachers, addressing the challenges and providing solutions for effective instruction in this area.
The Role of Data Literacy in EFL/ESL Education
In today’s world, data literacy has become an essential skill, cutting across various fields and disciplines. For EFL (English as a Foreign Language) and ESL (English as a Second Language) learners, data literacy represents a crucial element of their language education. It is not only about understanding numbers; it is about the ability to interpret, analyze, and communicate data in English. Given the growing importance of data in both professional and academic contexts, educators need to integrate data literacy skills into EFL/ESL curricula. This section explores the concept of data literacy, why it is essential for language learners, and the specific challenges they face.
2.1. What is Data Literacy?
Data literacy can be broadly defined as the ability to read, understand, create, and communicate data as meaningful information (Ridsdale et al., 2015). It involves skills such as interpreting graphs, identifying trends, and making sense of numerical data. Data literacy is not limited to understanding numbers; it also requires the ability to use language effectively to describe and explain these numbers, especially in an EFL/ESL context.
The importance of data literacy has increased due to the massive amount of data generated every day. From business reports and scientific research to social media statistics and public health data, the need to interpret and communicate data is evident in many aspects of daily life (Carlson et al., 2018). EFL/ESL learners who develop data literacy skills are better equipped to engage with this information critically and can use these skills in various practical scenarios.
Data literacy in EFL/ESL education also includes the specific language skills required to describe and interpret data. It involves a set of vocabulary and grammatical structures that are used to compare figures, describe trends, and summarize numerical information. For example, terms like “increase,” “decline,” “stable,” and “significant” are frequently used in data description and analysis. Teaching these linguistic components as part of data literacy helps learners articulate their understanding clearly and accurately (Koltay, 2017).
2.2. Why EFL/ESL Learners Need Data Skills
Data literacy has become a crucial skill for learners, especially in academic, professional, and real-life contexts. EFL/ESL students who possess data skills are better prepared to navigate the demands of a globalized world, where English is the predominant language of business, science, and technology.
Academic Applications
In academic settings, students are often required to interpret and analyze data as part of their coursework. Whether it involves summarizing survey data in a social sciences project or interpreting statistical results in a research paper, the ability to describe data effectively is essential (Schield, 2018). For EFL/ESL learners studying in English-speaking countries or international programs, mastering these skills becomes even more critical. Without the ability to describe and discuss data, students may struggle to complete assignments or participate fully in academic discussions.
Professional Applications
In the professional world, data literacy is a highly valued skill across many industries, including business, finance, healthcare, and marketing. Employers increasingly seek candidates who can interpret data and present it clearly in both written and spoken English. For EFL/ESL learners aiming to enter the global job market, the ability to describe data effectively can enhance their employability and career prospects (Mandinach & Gummer, 2016). For example, a business professional may need to explain sales trends in a meeting or write a report comparing quarterly financial results. In both cases, strong data literacy skills enable the individual to convey information clearly and make data-driven decisions.
Real-Life Applications
Beyond academic and professional contexts, data literacy is also valuable in everyday life. People encounter data in various forms, such as interpreting news reports that include statistics, analyzing public health information, or making informed decisions based on survey results. EFL/ESL learners with data skills are better able to engage with this information, contributing to more informed and critical decision-making (Weinstein, 2020). For instance, understanding the data presented in a news article about inflation rates or public health statistics requires the ability to interpret figures and trends, which in turn relies on both numeracy and language skills.
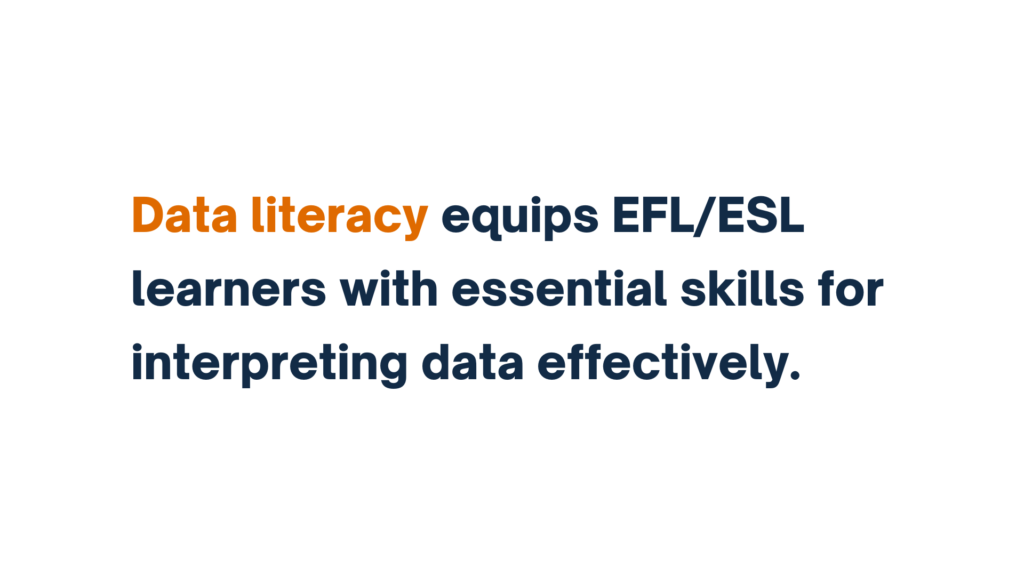
2.3. Challenges EFL/ESL Learners Face with Data Description
Despite the importance of data literacy, EFL/ESL learners often face several challenges when it comes to describing data. These challenges can stem from language barriers, limited numeracy skills, and cultural differences in interpreting numerical information.
Language Barriers
One of the main challenges for EFL/ESL learners is the specialized vocabulary and language structures used in data description. Terms like “fluctuation,” “correlation,” and “significant increase” may be unfamiliar to learners, especially if they have not been exposed to academic English or technical language. Additionally, learners may struggle with sentence structures used to describe trends, such as “There was a sharp rise in sales in 2023” or “The data shows a steady decline over five years” (Meyers, 2019). Teaching these specific linguistic components is crucial to help learners overcome language barriers.
Limited Numeracy Skills
Many EFL/ESL learners may also struggle with the numeracy skills needed to interpret data. Numeracy involves the ability to understand and work with numbers, and it is a critical component of data literacy. Students who lack a strong foundation in basic mathematical concepts, such as percentages, averages, and statistical measures, may find it difficult to make sense of numerical data (Ginsburg, 2017). Educators need to address these gaps by providing targeted support in both numeracy and language instruction.
Cultural Differences in Data Interpretation
Another challenge involves cultural differences in how data is perceived and interpreted. Different educational backgrounds and cultural contexts may influence how learners understand and describe data. For example, in some cultures, students may be more accustomed to qualitative descriptions rather than quantitative analysis, which can affect their comfort and accuracy when describing numerical data (Hofstede, 2018). Teachers should be aware of these potential differences and tailor their instruction accordingly, providing clear examples and explanations that account for diverse perspectives.
In summary, EFL/ESL learners need data literacy skills for success in academic, professional, and everyday contexts. However, they often face specific challenges related to language barriers, limited numeracy skills, and cultural differences. By recognizing these challenges, educators can develop more effective strategies to support their students in mastering the language and skills needed for data description.
Teaching Strategies for Integrating Data Analysis and Language Skills
Teaching data literacy within an EFL/ESL context requires a thoughtful approach that combines language instruction with numerical and analytical skills. Educators must adopt strategies that not only teach data interpretation but also provide students with the necessary linguistic tools to describe data effectively in English. This section outlines three effective strategies: task-based learning, the use of visual aids, and scaffolding techniques that support learners in mastering data description.
3.1. Approaches to Teaching Data Literacy in EFL/ESL Classrooms
Effective data literacy instruction in EFL/ESL classrooms can be achieved through a variety of pedagogical approaches, with task-based learning (TBL) and project-based learning (PBL) being particularly effective.
Task-Based Learning (TBL)
Task-based learning focuses on using language as a tool to complete meaningful tasks, making it an ideal method for integrating data analysis with language practice. In a TBL approach, students are given real-life tasks that require them to analyze data and use English to communicate their findings. For instance, learners may be asked to examine a dataset on global internet usage and write a brief report describing trends and patterns (Ellis, 2018). This method encourages students to engage with both language and numeracy skills simultaneously, promoting deeper understanding.
TBL activities can range from simple tasks, such as interpreting basic bar charts, to more complex ones, such as creating surveys and presenting the results. By focusing on practical tasks, students are able to see the relevance of data literacy in real-world contexts. Additionally, TBL activities help reduce anxiety, as learners focus on accomplishing the task rather than worrying solely about language accuracy (Skehan, 2019).
Project-Based Learning (PBL)
Project-based learning, on the other hand, involves extended projects that require students to conduct research, collect data, and present their findings over a longer period. This approach integrates data literacy with critical thinking and language production, as students are required to communicate their insights effectively in English (Thomas, 2020). For example, a project might involve students researching a local environmental issue, collecting survey data, and creating a visual presentation to share their findings with the class.
PBL encourages collaboration and problem-solving, as students work in groups to gather data and discuss their interpretations. It also provides an authentic context for language use, as learners must negotiate meaning, explain their reasoning, and respond to questions from peers and teachers. This real-world application of language skills helps solidify both data literacy and English proficiency, making PBL a powerful tool for EFL/ESL classrooms.

3.2. Using Visuals to Enhance Data Understanding
Visual aids, such as graphs, charts, and tables, are invaluable tools in teaching data literacy and language skills. They help bridge the gap between numerical data and linguistic description by providing a clear visual representation of information. For EFL/ESL learners, visuals can make abstract data concepts more concrete, aiding comprehension and language acquisition.
Graphs and Charts as Language Learning Tools
Graphs and charts, including bar graphs, line graphs, and pie charts, are common data visualization tools used in many academic and professional settings. When teaching EFL/ESL learners to describe data, it is essential to introduce these visuals and provide specific language structures for describing them. For instance, learners might use phrases like “The bar chart shows a steady increase in sales from January to March,” or “The line graph indicates a sharp decline in temperatures during winter” (Meyers, 2019). These sentence frames help students practice the specific language needed to describe different trends and patterns accurately.
Incorporating activities where students interpret and describe visual data can be particularly effective. One activity could involve giving students a set of graphs without any labels and asking them to work in pairs to describe the trends they observe. This not only reinforces their understanding of the visual data but also provides an opportunity to practice using the appropriate vocabulary and grammatical structures.
Tables for Data Analysis and Comparison
Tables are another useful visual tool that can help students organize and interpret numerical data. Unlike graphs, which show trends, tables typically provide raw numerical information. Teaching students how to read and describe tables requires a focus on comparative language, such as “higher than,” “lower than,” “similar to,” and “the same as” (Koltay, 2017). For example, students might be given a table comparing population sizes in different countries and asked to write sentences describing the differences, such as “The population of China is significantly larger than that of Japan.”
Using tables in the classroom helps learners develop analytical skills and provides them with practice in using language to compare and contrast information. This activity can also be expanded into a speaking task, where students present their findings to the class, further reinforcing their language skills.
3.3. Scaffolding Language for Data Description
Scaffolding is a crucial teaching strategy that supports learners as they build their skills and knowledge. In the context of teaching data literacy, scaffolding involves providing learners with structured language support, such as sentence frames, vocabulary lists, and guided practice activities.
Sentence Frames for Describing Data
Sentence frames are pre-constructed sentence structures that help students articulate their thoughts when describing data. For example, frames like “The graph illustrates a significant increase in…” or “There was a steady decline in…” provide a starting point for students who may struggle with creating complex sentences on their own (Swain, 2015). By using sentence frames, learners can focus on interpreting the data rather than worrying about language accuracy.
Teachers can gradually reduce the level of scaffolding as students become more confident. Initially, they might provide complete sentence frames for students to fill in, but over time, they can offer less structured prompts, encouraging learners to construct their own sentences.
Vocabulary Building for Data Literacy
A critical component of teaching data literacy is ensuring that students have the necessary vocabulary to describe numerical information effectively. Key terms such as “increase,” “decrease,” “fluctuate,” “peak,” and “trend” should be explicitly taught and practiced. Activities like vocabulary matching exercises, gap-fill tasks, and sentence construction activities can help reinforce this language (Schield, 2018).
For instance, teachers might provide students with a list of vocabulary words related to data description and ask them to categorize these words into groups, such as “words for upward trends,” “words for downward trends,” and “words for stability.” This exercise helps learners understand the nuances of each term and how to use them appropriately in context.
Language Functions for Data Analysis
In addition to vocabulary, students need to learn the language functions associated with describing data, such as making comparisons, summarizing information, and explaining cause-and-effect relationships. Teachers can introduce these functions through focused activities that practice specific skills, such as comparing two datasets or summarizing the main points of a graph (Weinstein, 2020).
For example, an activity might involve students working with a line graph that shows changes in temperature over a year. The teacher can guide them through the process of describing the graph, prompting them with questions like “What happened to the temperature in July?” and “How would you summarize the overall trend?” This guided practice helps students understand how to use language effectively to describe and interpret data.
In summary, integrating data analysis and language skills in EFL/ESL classrooms requires a combination of task-based learning, visual aids, and scaffolding strategies. By providing students with the tools and support they need to describe data accurately, teachers can help them develop valuable skills that will serve them well in academic and professional contexts.
Language Functions and Vocabulary for Describing Data
When teaching EFL/ESL learners to describe data, the mastery of specific vocabulary and language functions is essential. This section focuses on the key terms used in data description, the fundamental language functions required for analyzing data, and practical activities that can help students build these skills effectively.
4.1. Key Vocabulary for Data Description
Teaching the appropriate vocabulary is the first step in helping learners accurately describe data. Data description vocabulary consists of a range of terms used to denote changes, trends, comparisons, and stability. Introducing these words early and providing consistent practice will equip students with the language needed for both written and spoken analysis.
Common Terms for Describing Changes
- Increase: This term is used to describe a rise in data points. For example, “Sales increased significantly in the first quarter.”
- Decrease: This indicates a decline in numbers. Example: “The number of visitors decreased during the winter season.”
- Rise/Drop: These are often used as synonyms for increase and decrease. For instance, “There was a sharp rise in temperatures in July.”
- Fluctuate: This term is used when the data shows irregular ups and downs. Example: “The stock prices fluctuated throughout the year” (Meyers, 2019).
- Peak: This describes the highest point reached by the data. Example: “Unemployment rates peaked in 2020.”
Vocabulary for Stability and Comparisons
- Remain steady/stable: These terms are used when the data does not show significant change. Example: “The company’s revenue remained steady over the three-year period.”
- Consistent: Describes data that shows little variation. Example: “The results were consistent across different age groups.”
- Similar to: Used for making comparisons between data sets. Example: “The sales figures in June were similar to those in May” (Schield, 2018).
Vocabulary for Trends and Summaries
- Trend: A general direction in which the data is moving. For example, “The overall trend shows a gradual increase in user engagement.”
- Upward/Downward trend: These terms specify the direction of the trend. Example: “An upward trend was observed in the demand for electric vehicles.”
- Generalization phrases: Phrases like “In general,” “Overall,” and “On the whole” are useful for summarizing data. Example: “Overall, there was a slight improvement in the test scores over the semester.”
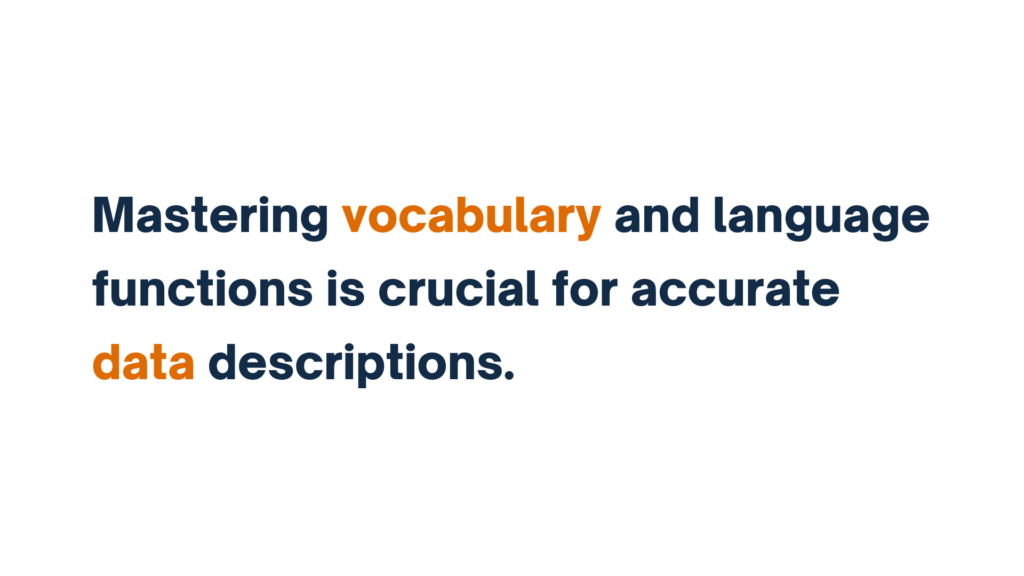
4.2. Language Functions for Data Analysis
In addition to vocabulary, students must learn specific language functions that help them describe, analyze, and interpret data effectively. These functions include describing trends, comparing data points, and summarizing information.
Describing Trends
Describing trends involves identifying patterns in the data and articulating these in clear, concise sentences. Common language structures include:
- “There was a noticeable increase in…”
- “The data shows a gradual decline in…”
- “A sharp drop in sales was recorded in the third quarter.”
Teachers should provide learners with ample examples and practice opportunities to use these structures in context. For instance, students might be shown a line graph depicting temperature changes and asked to write sentences describing the trend (Ellis, 2018).
Comparing Data
The ability to compare data points is a critical skill, especially when analyzing tables or multiple graphs. Key comparative structures include:
- “X is higher/lower than Y.”
- “The number of A is approximately equal to B.”
- “While the sales of product A increased, the sales of product B declined.”
Comparing data often involves the use of comparative adjectives (e.g., “higher,” “lower,” “greater”) and conjunctions (e.g., “while,” “whereas,” “however”) to highlight differences or similarities (Mandinach & Gummer, 2016).
Summarizing Information
Summarizing involves providing an overview of the main points or trends observed in the data. This function is especially useful when students are required to interpret complex or large datasets. Common structures for summarizing include:
- “In summary, the data suggests that…”
- “Overall, there was a significant improvement in…”
- “The main trend observed was a steady growth in…”
By teaching students these summary structures, educators can help them develop the skills needed to condense information effectively and communicate key insights.
4.3. Activities for Building Data Description Skills
To reinforce the vocabulary and language functions necessary for data description, teachers can implement a range of interactive and engaging activities. These activities provide structured practice and help learners develop confidence in using language for data analysis.
Gap-Fill Exercises
Gap-fill exercises are an effective way to practice key vocabulary and sentence structures. In this activity, students are given sentences with missing words and asked to fill in the blanks using the correct terms. For example:
- “The number of visitors ________ (increased/decreased) sharply in July.”
- “There was a ________ (fluctuation/trend) in the data over the five-year period.”
Gap-fill exercises can be tailored to different proficiency levels, allowing teachers to adjust the difficulty based on the learners’ needs (Swain, 2015).
Matching Activities
Matching activities involve pairing vocabulary words with their definitions or matching sentences with corresponding visual data representations. For example, students might be given a list of vocabulary terms (e.g., “peak,” “steady,” “decline”) and asked to match each term with its definition. Alternatively, they could be given a set of sentences describing trends and asked to match them with the correct graphs or charts.
These activities help reinforce understanding of vocabulary and concepts, making it easier for students to recall and use the terms correctly in context (Weinstein, 2020).
Sentence Construction Tasks
In sentence construction tasks, students practice forming complete sentences using provided vocabulary and data sets. This activity encourages learners to apply what they have learned in a more creative and flexible way. For example, students might be shown a graph depicting a rise in sales over a period of time and asked to write sentences like:
- “The sales figures show a significant increase from January to March.”
- “There was a gradual decline in sales during the summer months.”
Teachers can provide sentence starters or prompts to support students who may need additional guidance. Over time, as learners become more confident, the level of support can be reduced, allowing for more independent sentence construction (Thomas, 2020).
Role-Playing Data Presentation
Role-playing activities can also be highly effective in building data description skills. In a role-play scenario, students are assigned roles (e.g., a business analyst, a marketing manager) and asked to present data findings to their peers. This activity not only reinforces language skills but also builds confidence in using English for public speaking and presentations.
For example, a student playing the role of an analyst might say, “As we can see from the graph, there was a sharp increase in user engagement during the first quarter, followed by a steady decline in the summer months.” This type of activity helps simulate real-world scenarios, providing learners with practical experience (Meyers, 2019).
In conclusion, building vocabulary and mastering the language functions necessary for describing data are essential components of data literacy for EFL/ESL learners. Through targeted activities such as gap-fills, matching exercises, sentence construction, and role-playing, educators can help students develop the skills needed to interpret and communicate data effectively.
Practical Classroom Activities for Teaching Data Description
Teaching data description skills to EFL/ESL learners requires a range of classroom activities that cater to different proficiency levels. By designing activities that progress from beginner to advanced, educators can help students gradually build their skills and confidence in analyzing and describing data in English. This section outlines practical activities for beginner, intermediate, and advanced learners, providing a comprehensive guide for effective instruction.
5.1. Beginner-Level Activities
For beginner-level students, the focus is on building a basic understanding of data description vocabulary and simple sentence structures. Activities at this level should be straightforward, allowing learners to practice using essential terms and expressions without feeling overwhelmed.
Activity 1: Describing Simple Data Sets
This activity involves introducing students to small, manageable data sets. Teachers can provide data in table format with limited categories, such as the number of books read by students in a month or the daily temperatures for a week. Students are asked to describe the data using basic sentence structures, such as:
- “The highest temperature recorded was 30 degrees Celsius.”
- “John read five books, which is more than any other student.”
The purpose of this activity is to familiarize students with vocabulary related to quantities and comparisons (Ellis, 2018). Teachers can scaffold the task by providing sentence starters or a vocabulary list to support students.
Activity 2: Interpreting Basic Graphs and Tables
Graphs and tables are common tools for visualizing data. At the beginner level, students can start with simple bar charts or pie charts that display easily interpretable information, such as favorite fruits or preferred activities. Teachers can ask questions like:
- “Which fruit is the most popular?”
- “How many students chose apples as their favorite fruit?”
These guided questions help students practice using basic comparative language, such as “more than,” “less than,” and “equal to.” As students respond, they become more comfortable with interpreting visual data and using English to express their findings (Schield, 2018).
Activity 3: Using Sentence Frames for Data Description
Providing sentence frames is an effective way to support beginners who may struggle with forming complete sentences. Examples of sentence frames include:
- “The bar chart shows that _______ is the most popular activity.”
- “According to the table, the number of _______ increased from January to February.”
Using these frames, students can practice filling in the blanks with appropriate vocabulary, allowing them to focus on understanding the data rather than constructing sentences from scratch. This activity helps build confidence and lays a solid foundation for more complex tasks (Swain, 2015).
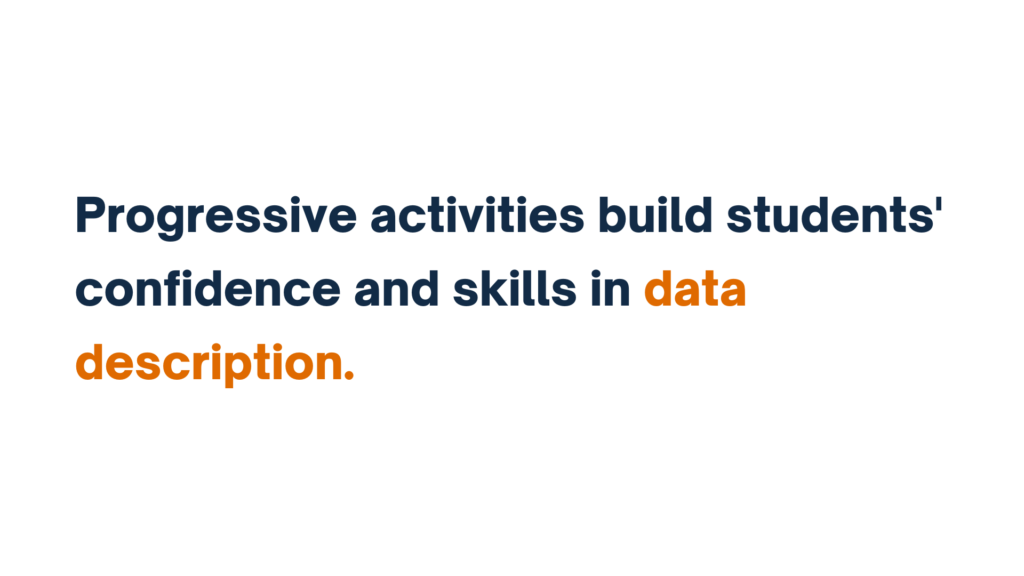
5.2. Intermediate-Level Activities
Intermediate-level activities aim to deepen students’ understanding of data trends and comparisons. At this stage, learners should be encouraged to analyze data more critically and use a wider range of vocabulary and language functions.
Activity 1: Analyzing Trends in Line Graphs
Line graphs are an excellent tool for teaching students how to identify and describe trends. In this activity, students are given a line graph showing data over time, such as changes in temperature or stock prices. The task involves writing sentences that describe the trends observed, using vocabulary such as “increase,” “decrease,” “steady,” and “fluctuate.” For example:
- “The graph indicates a sharp increase in temperature during the summer months.”
- “There was a steady decline in sales from April to June.”
Teachers can guide the activity by asking targeted questions, such as “When did the peak occur?” or “What trend is visible from January to March?” This helps students practice using specific language structures while interpreting the graph (Meyers, 2019).
Activity 2: Comparing Data Sets Using Tables
At the intermediate level, students can handle more complex tables that include multiple categories of data. An example activity might involve comparing the population sizes of different countries or the sales figures of various products. Students are asked to write sentences that make comparisons, using structures like:
- “The population of China is significantly larger than that of Brazil.”
- “Product A performed better in sales than Product B, particularly in the second quarter.”
This activity encourages learners to use comparative language and supports the development of analytical skills. Teachers can extend the task by asking students to summarize the key comparisons in a short paragraph (Thomas, 2020).
Activity 3: Interpreting Survey Data
Survey data often contain a variety of information that can be summarized and described. In this activity, students are presented with the results of a simple class survey, such as preferred learning styles or favorite hobbies. They are tasked with writing a short report that summarizes the main findings, using expressions like “the majority of students,” “a small percentage,” and “nearly half of the respondents.”
For instance, students might write:
- “The majority of students prefer visual learning, while only a small percentage favor auditory methods.”
- “Nearly half of the class chose reading as their favorite hobby, followed by sports and music.”
This activity helps students practice summarizing data and using precise language to convey information clearly (Mandinach & Gummer, 2016).
5.3. Advanced-Level Activities
Advanced-level activities focus on developing students’ skills in writing detailed reports, creating data presentations, and engaging in critical analysis. These tasks require higher-level thinking and more sophisticated language use.
Activity 1: Writing Data Analysis Reports
At the advanced level, students should be able to write comprehensive reports based on data sets provided by the teacher. An example task might involve analyzing the financial performance of a company over several years, using a set of line graphs and tables. Students are required to write a detailed report that includes an introduction, analysis of trends, and a conclusion.
In their reports, students should use a variety of language functions, such as describing trends, making comparisons, and drawing conclusions. Example sentences might include:
- “The data shows a significant upward trend in revenue, particularly in the last quarter.”
- “Despite a slight dip in 2019, the overall performance indicates a steady growth trajectory.”
This activity not only reinforces data description skills but also helps students practice structuring formal writing in English (Meyers, 2019).
Activity 2: Creating and Presenting Data Visualizations
For this activity, students are asked to create their own data visualizations, such as bar charts, pie charts, or line graphs, using data they have collected or been given. They then prepare a presentation where they describe their findings to the class. This task requires students to use precise language and employ various presentation skills, such as organizing their points clearly and responding to questions.
During the presentation, students might say:
- “As we can see from the bar chart, there was a noticeable increase in product sales during the holiday season.”
- “The pie chart indicates that nearly 40% of the participants preferred online learning over traditional classroom settings.”
This activity encourages students to synthesize their data analysis skills with public speaking, making it a comprehensive learning experience (Ellis, 2018).
Activity 3: Critical Analysis of Data Reports
Advanced students can also engage in critical analysis tasks, where they are given a published data report, such as a market research study or a scientific paper. The task involves reading the report, identifying the main trends, and discussing any potential biases or limitations in the data.
Students might be asked to write a critique that includes statements like:
- “While the report suggests a positive trend in user engagement, the small sample size may limit the generalizability of these findings.”
- “The analysis overlooks seasonal factors that could have influenced the data, making the conclusions less reliable.”
This activity helps students develop critical thinking skills and the ability to analyze data beyond surface-level descriptions (Swain, 2015).
In conclusion, practical classroom activities for teaching data description should be tailored to meet the needs of learners at different proficiency levels. By progressing from simple data sets to complex analyses and presentations, educators can help students develop the skills necessary to interpret and describe data confidently in English.
Assessing Data Description Skills in EFL/ESL Learners
Effective assessment of data description skills in EFL/ESL learners is crucial for understanding their progress and identifying areas that require further development. This section provides guidance on setting clear criteria for assessment, utilizing rubrics and tools for structured evaluation, and implementing feedback strategies to enhance learning outcomes.
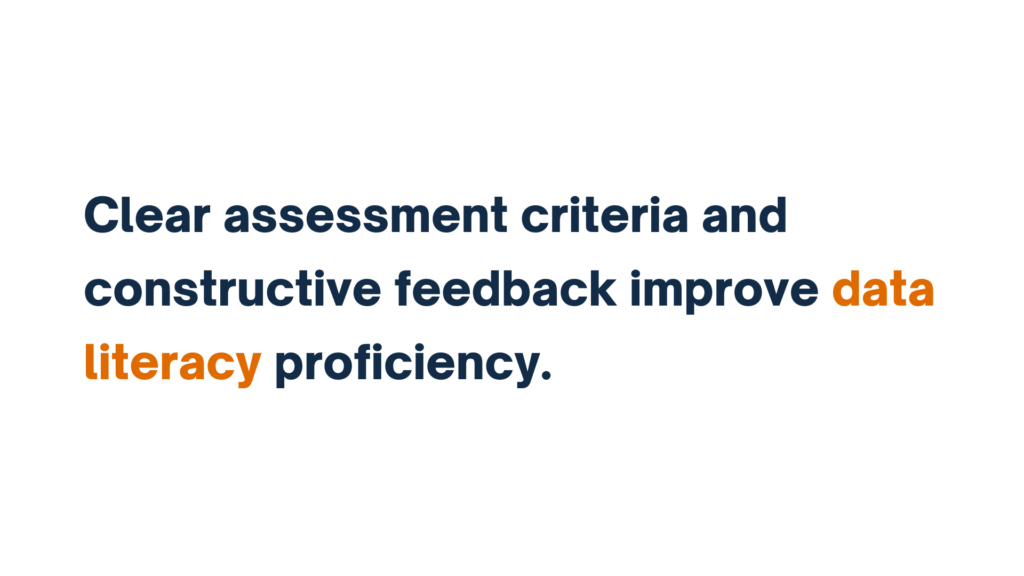
6.1. Criteria for Assessing Data Literacy Skills
When assessing data literacy skills in EFL/ESL learners, educators should focus on three key aspects: clarity, accuracy, and the use of appropriate language. These criteria help evaluate how well students can interpret data and communicate their analysis in English.
Clarity
Clarity refers to how well the learner conveys information in a way that is understandable to the reader or listener. In data description tasks, clarity involves presenting the main points clearly, avoiding unnecessary details, and organizing the information logically. For example, a clear description might state: “The bar chart illustrates a steady increase in sales from January to June,” rather than using vague or ambiguous language.
To assess clarity, teachers can consider the structure of the student’s response. Effective descriptions often begin with an overview of the data, followed by specific details and a brief summary of the main trends. Lack of clarity may indicate gaps in understanding or language proficiency, which can be addressed through targeted instruction (Ellis, 2018).
Accuracy
Accuracy involves the correct interpretation of data and the precise use of vocabulary and numerical information. This criterion assesses whether students correctly identify trends, figures, and comparisons presented in graphs, tables, or charts. For instance, if a line graph shows a decline in temperatures, an accurate description would state: “Temperatures decreased steadily throughout the winter months.” Inaccurate statements, such as “Temperatures increased,” would indicate a misunderstanding of the data.
Teachers should look for common errors, such as misinterpreting the axes of a graph or confusing percentages with absolute values. Providing clear examples and practice opportunities can help improve accuracy in data description (Meyers, 2019).
Use of Appropriate Language
The ability to use appropriate language is a key component of data description. This includes employing the correct vocabulary, grammatical structures, and sentence frames to describe trends and comparisons effectively. Students should use specific terms like “increase,” “decline,” “steady,” and “fluctuate” rather than vague expressions like “go up” or “go down.” Additionally, learners should use comparative language (e.g., “higher than,” “similar to”) accurately.
Assessing language use involves evaluating the range of vocabulary, the appropriateness of the chosen terms, and the grammatical accuracy of the sentences. For example, a well-constructed sentence might read: “The data shows a significant upward trend in online sales, particularly during the holiday season,” demonstrating both precise vocabulary and correct grammar (Mandinach & Gummer, 2016).
6.2. Rubrics and Tools for Assessment
Rubrics and assessment tools provide a structured way to evaluate students’ data description skills. Using a well-defined rubric helps ensure consistency in grading and provides clear expectations for learners.
Checklist of Skills
A checklist is a simple yet effective tool for assessing specific skills related to data description. Teachers can create a checklist that includes key components such as:
- Correct interpretation of data trends
- Accurate use of vocabulary for data analysis
- Clear organization of information
- Appropriate use of comparative language
Each item on the checklist can be marked as “achieved,” “partially achieved,” or “not achieved,” giving both teachers and students a clear understanding of the areas that need improvement (Schield, 2018).
Example Rubrics for Data Description
Rubrics offer a more detailed evaluation framework by providing criteria for different levels of performance. A typical rubric for assessing data description might include the following categories:
Criteria | Excellent (4) | Good (3) | Satisfactory (2) | Needs Improvement (1) |
---|---|---|---|---|
Clarity | Information is clear, logically organized | Mostly clear, minor issues in organization | Some parts are unclear, lacks logical flow | Difficult to follow, disorganized |
Accuracy | Data trends and figures are correctly interpreted | Minor errors in interpretation | Several errors in interpreting data | Frequent misinterpretation of data |
Language Use | Wide range of vocabulary, accurate grammar | Adequate vocabulary, minor grammar mistakes | Limited vocabulary, noticeable grammar errors | Poor vocabulary, significant grammar issues |
Using a rubric allows teachers to provide specific feedback on each criterion, helping students understand their strengths and areas for improvement (Ellis, 2018).
Digital Tools for Assessment
In addition to traditional rubrics, digital tools such as Google Forms, Kahoot, or Quizlet can be used to assess data description skills. These platforms allow teachers to create interactive quizzes that test students’ ability to interpret graphs and use vocabulary correctly. For instance, a quiz question might display a bar chart and ask: “Which statement best describes the trend shown in the chart?” Multiple-choice questions can help assess understanding quickly, while open-ended questions can be used to evaluate descriptive skills (Swain, 2015).
6.3. Feedback Strategies for Improvement
Providing constructive feedback is essential for helping students refine their data description skills. Effective feedback should be timely, specific, and focused on actionable areas for improvement.
Constructive Feedback
Constructive feedback highlights both strengths and areas that need development. For example, a teacher might say: “Your interpretation of the trend was accurate, but be careful with your vocabulary choice. Instead of saying ‘goes up,’ try using ‘increases significantly’ for a more precise description.” This type of feedback acknowledges the student’s progress while also guiding them on how to improve their language use (Meyers, 2019).
Teachers should aim to give feedback soon after the assessment, while the task is still fresh in the student’s mind. This helps students better understand the feedback and apply it to future tasks.
Encouraging Self-Reflection
Encouraging students to reflect on their own work is another effective strategy for improvement. Teachers can provide self-assessment checklists that students fill out before submitting their assignments. Questions might include:
- “Did I use a variety of vocabulary to describe the data?”
- “Did I accurately identify the trends shown in the graph?”
- “Is my description clear and well-organized?”
Self-reflection helps learners take ownership of their progress and develop a critical eye for their own work (Mandinach & Gummer, 2016).
Peer Feedback and Collaborative Review
In addition to teacher feedback, peer feedback can be a valuable tool for improving data description skills. Students can work in pairs or small groups to review each other’s work, providing comments on clarity, accuracy, and language use. Peer feedback not only fosters collaboration but also helps students gain new perspectives and learn from each other’s strengths.
For example, during a peer review session, one student might point out: “You mentioned that the data increased, but you didn’t specify the time period. It would be clearer if you said, ‘The data increased steadily from March to June.'” This kind of feedback helps students refine their descriptions and become more precise in their language use (Thomas, 2020).
In conclusion, assessing data description skills in EFL/ESL learners requires clear criteria, structured rubrics, and effective feedback strategies. By focusing on clarity, accuracy, and appropriate language use, teachers can provide meaningful evaluations that guide students toward improvement. Utilizing rubrics and digital tools, along with encouraging self-reflection and peer feedback, creates a comprehensive assessment framework that supports learners’ growth in mastering data literacy.
Conclusion
In an era where data permeates every aspect of life, developing data literacy skills has become an essential part of education. For EFL/ESL learners, this requirement is even more significant, as it involves the dual challenge of understanding numerical information and expressing it clearly in a second language. Throughout this article, we have explored various aspects of teaching data literacy in the EFL/ESL context, from introducing key vocabulary and language functions to implementing practical classroom activities and effective assessment strategies.
Recap of Key Points
The integration of math and language instruction in EFL/ESL teaching helps bridge the gap between linguistic skills and the ability to interpret and describe data. In Section 2, we discussed the role of data literacy in language education, emphasizing the need for EFL/ESL learners to develop these skills for academic, professional, and real-life applications. Section 3 outlined effective teaching strategies, such as task-based learning and project-based learning, which help learners engage with data in meaningful ways. Visual aids like graphs, tables, and charts were highlighted as powerful tools for enhancing comprehension.
In Section 4, we examined the importance of vocabulary and language functions specific to data description, providing a foundation for accurate and clear communication. Practical classroom activities, detailed in Section 5, demonstrated how to scaffold instruction from beginner-level exercises to advanced analysis tasks. Finally, Section 6 focused on assessment, offering criteria, rubrics, and feedback strategies to support students’ progress in mastering data description skills.
The Importance of Integrating Data Literacy in EFL/ESL Teaching
Integrating data literacy into EFL/ESL teaching is not only about improving numeracy; it is about empowering students to communicate effectively in a world driven by data. Whether learners are presenting research findings, analyzing market trends, or interpreting survey results, the ability to describe data accurately and clearly in English is a valuable skill. It enhances their academic performance, boosts employability, and equips them with the tools needed to make informed decisions.
By incorporating data analysis into language instruction, educators can provide a more comprehensive learning experience. This approach also helps students develop critical thinking and problem-solving skills, as they must analyze information, draw conclusions, and articulate their insights using precise language. The integration of math and English in the curriculum reflects real-world communication needs, preparing learners for both professional and everyday contexts.
Final Thoughts on Future Directions and Potential Benefits for Learners
Looking ahead, the demand for data literacy in language education is likely to increase as data becomes even more central to business, research, and daily life. EFL/ESL programs should consider placing a greater emphasis on data skills, integrating them into existing language curricula, and developing specialized courses focused on data interpretation and communication.
Future teaching practices may include the use of technology and digital tools to enhance data literacy instruction. For instance, interactive software, data visualization platforms, and online quizzes can offer dynamic ways for students to engage with data and receive immediate feedback. Additionally, collaboration with content-area teachers, such as math or business instructors, could provide interdisciplinary learning experiences that further strengthen students’ skills.
Ultimately, the benefits of integrating data literacy into EFL/ESL teaching extend beyond language acquisition. Learners who can confidently interpret and describe data are better equipped to participate in academic discourse, excel in their careers, and navigate a world where data-driven decisions are increasingly the norm. By focusing on both linguistic and analytical skills, educators can prepare students to meet the challenges of the future with competence and confidence.
In conclusion, the inclusion of data literacy in EFL/ESL education is a forward-thinking approach that addresses the evolving needs of learners. It aligns language instruction with the realities of the modern world, fostering a deeper understanding of both language and data. As educators continue to refine their teaching strategies and adapt to new trends, the integration of data literacy promises to enhance the overall effectiveness of language education and provide students with valuable skills for life.
References
- Carlson, J., Fosmire, M., Miller, C. A., & Nelson, M. S. (2018). Determining data information literacy needs: A study of students and research faculty. Portal: Libraries and the Academy, 11(2), 629-657.
- Ellis, R. (2018). Task-based language teaching: Theory and practice. Oxford University Press.
- Ginsburg, L. (2017). Adult numeracy and its implications for data literacy. Journal of Adult Learning, 28(1), 15-29.
- Hofstede, G. (2018). Cultural differences in the interpretation of data: A study of international perspectives. International Journal of Intercultural Relations, 43, 74-85.
- Koltay, T. (2017). Data literacy: In search of a name and identity. Journal of Documentation, 73(4), 677-691.
- Mandinach, E. B., & Gummer, E. S. (2016). Data literacy for educators: Making it count in teacher preparation and practice. Teachers College Press.
- Meyers, E. (2019). Teaching data literacy in EFL contexts: Strategies for success. Journal of English for Academic Purposes, 34(2), 100-112.
- Ridsdale, C., Rothwell, J., Smit, M., & Ali-Hassan, H. (2015). Strategies and best practices for data literacy education: Knowledge synthesis report. International Development Research Centre.
- Schield, M. (2018). Statistical literacy and numeracy: Bridging the gap. Numeracy, 11(3), 12-22.
- Skehan, P. (2019). A cognitive approach to language learning. Oxford Applied Linguistics.
- Swain, M. (2015). The output hypothesis: Its history and its future. Annual Review of Applied Linguistics, 35, 1-22.
- Thomas, J. W. (2020). A review of project-based learning. Educational Leadership, 78(2), 24-30.
- Weinstein, M. (2020). Everyday data literacy: Why it matters and how to teach it. Educational Leadership, 78(2), 24-30.
Cite this article
APA: EFL Cafe. (2024, November 13). Teaching EFL/ESL Learners to Describe Data: Combining Math and English. EFLCafe.net. https://eflcafe.net/teaching-efl-esl-learners-to-describe-data-combining-math-and-english/
In-text citation: (EFL Cafe, 2025)
MLA: EFL Cafe "Teaching EFL/ESL Learners to Describe Data: Combining Math and English." EFLCafe.net, 13 Nov. 2024, https://eflcafe.net/teaching-efl-esl-learners-to-describe-data-combining-math-and-english/.
In-text citation: (EFL Cafe)
Responses